With rapid advancements in AI, a very significant shift in the financial sector is in store. A lot of ground for this development can be traced through the domain of bank statement analysis, typically a manual, laborious activity of data extraction, arrangement, and analysis from complex financial sources. AI in financial statement analysis uses technologies such as Machine Learning and Natural Language Processing to make the process faster and more accurate than traditional methods by automating the extraction of data, detection of patterns, and provision of insights. In addition to improving efficiency, it increases fraud detection and ensures more secure financial operations.
With organizations navigating their way through an increasingly data-driven financial ecosystem, the use of AI solutions to analyze bank statements has become strategic. This blog looks into the role of AI in shaping the future of financial intelligence, promoting smarter decision-making and operational excellence.
Definition of AI in Bank Statement Analysis
In the case of AI-driven bank statement analysis, data is automatically extracted and evaluated. This is an advanced method through which large transaction records are identified with patterns and insights by the application of ML techniques and AI algorithms. Categorizing transactions, sorting data in a fast way, and pinpointing anomalies that might be an irregularity indicative of fraudulent activity help AI systems speedily. Also, AI monitors spending trends and predicts future expenditures.
AI-based Bank Statement Analyzers revolutionize the way traditional data evaluation is done. It automates the entire process, scanning huge amounts of data in a matter of moments to reveal patterns, risks, and anomalies that would otherwise be missed in manual analysis. This results in the quick processing of loan approvals, high exposure to fraud detection, and better decision-making abilities for lenders and borrowers, resulting in better financial intelligence outcomes.
Key Features of AI-Driven Analysis
- Data Categorization: AI-powered software automatically recognizes and segments financial transactions into groups like revenue and expenses. This aids companies in competition, as trend analysis is employed to gain knowledge on financial intelligence and spending. Such advanced algorithms in NLP include key-value pair extraction methods for better categorization and reorganization of data.
- Data Visualization: AI-driven technologies convert intricate financial data into easily understood visual representations like charts and graphs. These visualizations enable better decision-making due to easy understanding of data. The AI system uses the prior data to generate reports comprehensive in showing trends and patterns.
- Credit Scoring: AI algorithms quicken the process of credit evaluation by considering records of past transactions. These models assist organizations in building exact risk profiles, considering creditworthiness, and producing valuable financial information, which helps in liquidity assessment along with gaining an understanding of market trends.
- Balance Sheet Audits: AI improves balance sheet audits’ precision and effectiveness. It follows the cash flow, reconciles discrepancies of finance, and ensures that all financial documents are scrutinized by providing facilities like text recognition and pattern analysis.
How AI Works in Bank Statement Analysis?
Processing both digital and physical account information is necessary to extract data from bank statements. The use of OCR technology can automate this process, which makes it easier by recording essential data that may include dates of transactions and amounts and descriptions.
It’s through the utilization of Machine Learning (ML) and Natural Language Processing (NLP) that Artificial Intelligence enhances the analysis of bank statements. These technologies are beneficial compared to the archaic manual procedures and systems, for they transform unprocessed financial data into useful insights and reduce errors and processing time. The core part of natural language processing is Named Entity Recognition (NER), which is essential because it successfully identifies and categorizes textual items such as beneficiary names and transaction details.
Step-by-Step Guide to Implementing AI
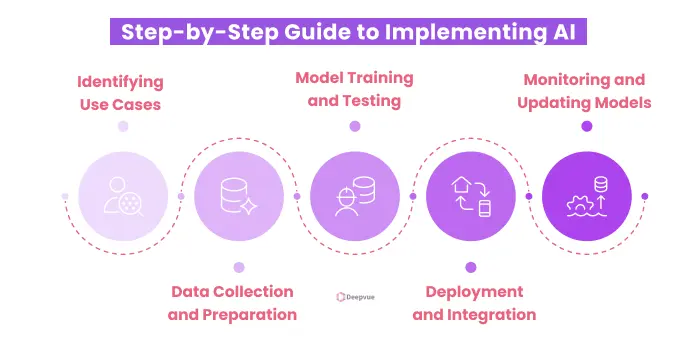
Stage 1: Identifying Use Cases
The first step in implementing AI for bank statement analysis is to analyze the types of use cases. Identify if it’s going to be used institutionally or by a personal end user. That means either assisting in the personal budgeting process for tracking expenses, or in institutions where the systems need fraud detection system upgrade or enhancement and even updating the customer’s credit scoring assessment.
Stage 2: Data Collection and Preparation
Data forms the backbone of any AI implementation. Analyze data from different types of customers who may be providing their data in different formats; PDFs, spreadsheet formats, or raw transaction logs. Look for and eliminate partially or wholly erroneous data input, while upholding data integrity and confidentiality. Correctly annotated and well-organized data is a must for training the AI models.
Stage 3: Model Training and Testing
Once the data is ready, training and testing of AI models are the focus. Based on the problem’s complexity and data characteristics, the selection of the models can be done. Pattern and trend discovery in customer transaction data would give insights to the predictive insights. Test the system intensively and evaluate model accuracy with diverse data sets, which can make it generalize well.
Stage 4: Deployment and Integration
Implement the AI model into the financial systems, and deploy it on suitable environments, depending on organizational needs, to be cloud-based for scalability or on-premise for tighter control. APIs will be created so that fluid exchange of data will be possible among systems. An interface should also be designed so that easy access with actionable insights is provided; however, strict security measures should be implemented to protect sensitive financial data.
Stage 5: Monitoring and Updating Models
The AI model needs continuous monitoring and improvement to be effective. Monitor model performance and track if it goes beyond the expected outcomes. Retrain models with new data from time to time and keep them updated. Ask for user feedback on emerging challenges and opportunities to improve. Update knowledge of the changing regulatory requirements and ensure that one is complying.
Best Practices for Implementing AI
- Use accurate, complete, and diverse datasets to train AI models.
- Clearly define objectives to guide tool selection and testing.
- Involve stakeholders from different departments to get their opinions and insights.
- Monitor model performance regularly and correct inaccuracies.
- Stay updated on industry trends and regulatory changes.
- Implement strong data security measures to safeguard sensitive information.
Benefits of AI in Banking
- Improving Customer Experiences: Customer service in the banking industry has changed as a result of the incorporation of AI-powered solutions. Virtual assistants and intelligent chatbots provide 24/7 support, enabling customers to access information and assistance whenever they need it. This level of timely service improves customer satisfaction and engagement.
- Increasing Regulatory Compliance: AI is a necessity for helping banking organizations navigate complex regulatory environments. AI reduces the time and cost of these complex processes by automating checks for compliance and generating detailed reports. Advanced AI systems can monitor multiple data sources to ensure compliance with privacy laws and AML procedures.
- Simplifying Risk Management: AI empowers banks with robust capabilities to proactively manage risk by processing massive amounts of data from numerous sources. Data streams culled from news stories, financial intelligence reports, and other streams can thus alert AI to new dangers, including possible legal challenges or reputational concerns to specific industries or businesses.
Challenges in Adopting AI Technologies
- Bias and Discrimination: AI models learn from historical data, which can often be biased. If these biases are not addressed carefully, AI systems can reinforce discrimination in decision-making processes, which may sometimes lead to unfair outcomes; for instance, people of a certain customer segment might not get credit, or transactions may be misclassified.
- Data Security and Customer Consent: AI systems collect huge amounts of sensitive customer information, which requires proper data safety and privacy arrangements. Unauthorized or breach of confidential customer information poses a threat to trust and penalization by authority regulations. Hence, obtaining correct and informed permission from customers toward data usage should be obtained correctly.
- High Cost: The adoption of AI technologies involves significant financial intelligence. Data infrastructure and model development and deployment are costs, and running is an additional expense. Therefore, small to medium-sized financial institutions may have trouble justifying such costs. One way out of the challenge would be exploring scalable cloud solutions, focusing on the use cases with the greatest return on investment.
Conclusion: The Future of AI in Financial Analysis
Artificially intelligent analysis of bank statements is changing the entire process of data computing and processing in the financial sectors. Along with improved accuracy levels and detection of unstructured data, AI enables a business to make the best financial decisions it can, as well as reduce operational risk. The future only promises more impressive capabilities in using AI to strengthen financial intelligence; it will also allow for better security, greater efficiency, and deeper insights into managing finances. Adopting bank statement analysis now will allow organizations to stand better in this data-driven future.
FAQ:
What is the future of artificial intelligence in banking?
The future of banking and AI implies more automation of the processes within banks, delivering more personalized services to customers and improved fraud detection. AI should help banks achieve smarter decision-making and make these institutions more streamlined, error-proof, and secure.
What is artificial intelligence in financial statement analysis?
AI in financial statement analysis applies technologies such as Machine Learning and Natural Language Processing to automate the extraction of data, detect patterns, and offer insights, thus making the process faster and more accurate than the traditional methods.
Can AI analyze bank statements?
Yes, AI can look at bank statements by extracting and organizing data regarding the amount, date, and description of a transaction. Accuracy is improved and processing is fastened, helping in the identification of anomalies.
How has AI impacted finance banking technology?
AI has transformed banking by automating processes, enhancing security, detecting fraud, enabling data-driven decision-making, improving efficiency, and reducing risks across the industry.
Why is it so important for banks to become “AI-first”?
Becoming “AI-first” is therefore an opportunity for banks to stay in the game while staying competitive with the advanced use of technologies that promise faster decision-making, quicker customer services, and enhanced operational efficiencies.