Different industries are given a due diligence mandate so that they depend on valid identity documents, contracts, and very critical documents to ensure security and compliance. The conventional manual verification methods are slow, error-prone, and unwilling to catch up with the acceleration and scale-up of pace.
The AI OCR is beyond the routine text extraction. It has combined artificial intelligence and machine learning, so it autonomously interprets, dissects, and validates documents accurately. Whether it is extracting data from passports, catching forged IDs, or verifying handwritten forms, OCR document verification makes easy the job with fewer errors and shorter turn-around times.
How Optical Character Recognition Works?
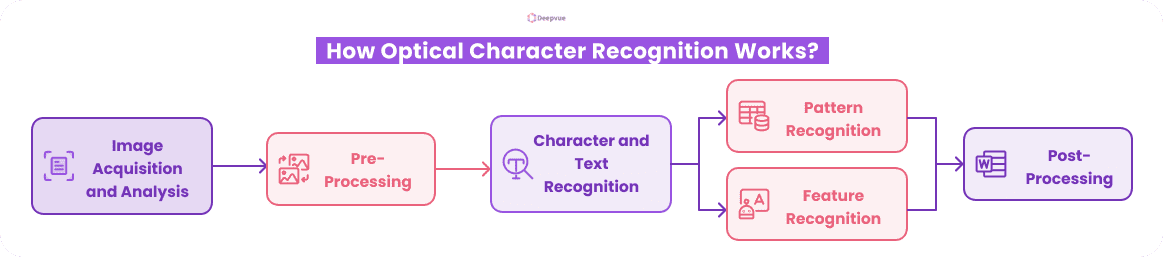
- Image Acquisition and Analysis: Image acquisition is one of the primary steps in the OCR document verification process. Here, a physical document or a text-based image is obtained based on the observed quality of the resultant image. After an image has been captured, it analyses issues related to blurriness, shadows, or lack of even lighting and tries to correct them.
- Pre-Processing: This step must be carried out with great care to make the image clean, clear, and suitable for accurate character recognition. The image captured is subjected to various techniques to remove distortions, correct for skewness, binarize, etc. to improve the quality of the image. By doing this, the OCR system can accurately recognize and extract text.
- Character and Text Recognition: It is one of the core steps in the OCR document verification. In this step, the system recognizes the segmented characters and converts them into machine-readable text. This is done primarily through two methods: pattern recognition, a comparative investigation between character shapes in a particular unmodified document against a defined database of character templates, and feature recognition, to assess and analyze certain properties of certain characters to identify them accurately.
- Post-Processing: This is the final stage of the OCR process, which verifies that the ultimate output is both visually and contextually consistent with the source content. This process includes methods such as spell-checking, formatting, grammar checking, and contextual analysis to check for accuracy and usability.
Benefits of AI-Powered OCR
- Enhanced Accuracy and Efficiency: The major advantage of OCR technology is that it transforms scanned documents, handwritten data, or images into machine-readable format. Companies can swiftly and accurately process large amounts of data, removing time-consuming manual data entry.
- Reduced Human Errors: All the ranges of typos, misreadings, or omissions that are subject to human entry errors are prevented by OCR technology. With its automation of document extraction and document verification, OCR technology is capable of providing enhanced accuracy in managing data.
- Regulatory Compliance: KYC and AML regulations must be adhered to by industries like banking, finance, or healthcare. With OCR technology, businesses can meet these regulatory requirements by automating and verifying the identities of their customers accurately and maintaining detailed reports.
- Fraud Prevention and Detection: OCR document verification can help to identify and prevent fraud. This is accomplished by identifying tampered or forged documents and establishing the authenticity of the identity documents. Businesses can incorporate OCR into their verification process to protect their operations and customers.
- Improved Customer Experience: A good customer experience is needed in terms of building trust and loyalty. The OCR technology can automate processes such as account opening, loan requests, or transaction approvals, thereby offering customers an enhanced and improved experience.
How Is OCR Software Used in Identity Verification?
IDV (Identity verification) is a core process applied in numerous industries. IDV’s primary purpose is to match the user-provided identity information with trustworthy sources such as government databases or credit bureaus. When AI OCR software combines with identity verification, it makes data extraction from identity documents such as passports, government IDs, etc., much more efficient.
When a user scans an image of his ID, the OCR software will instantly pull out information such as name, address, and DOB. The information will be cross-referenced with a trustworthy database to assess the legitimacy of the document. Furthermore, OCR document verification can examine the layout, fonts, and other security elements of the document to detect forged and tampered documents.
OCR is one of the indispensable tools used in identity verification, promoting accuracy, which in turn helps cut down processing time while providing an improved user experience. Also, OCR document verification cuts down friction during the onboarding or transaction process, letting companies ramp up the volumes efficiently.
Limitations of Traditional OCR
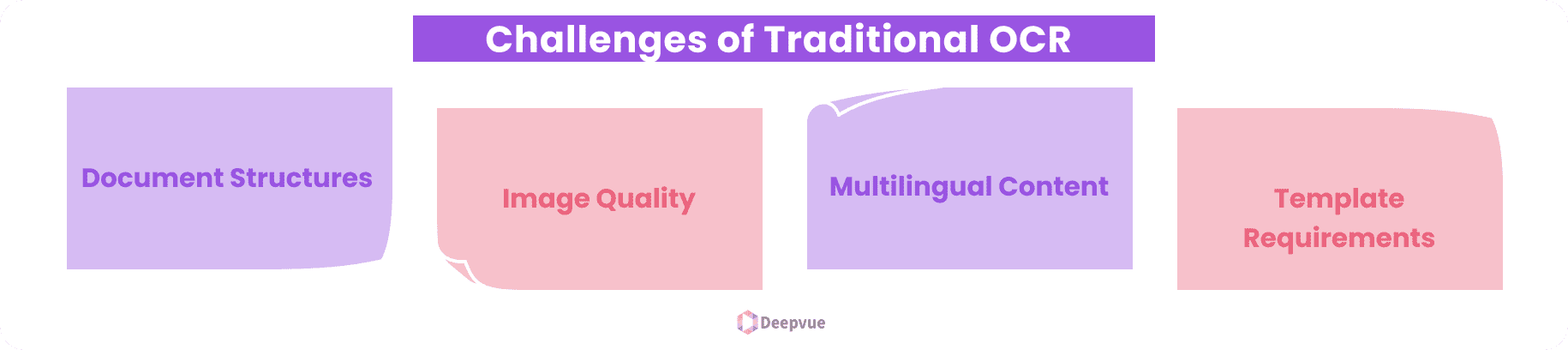
- Complex Document Structures and Layouts: Template-based OCR systems need to have predefined document types, dimensions, and alignment specifications to work properly. Although they work well with standardized layouts, they are not able to handle unusual or complex documents, including those with complex tables, multiple columns, or embedded images.
- Image Quality Problems: The performance of template-based OCR relies very much on the quality of the input images. Scans at low resolution, distorted text, or insufficient lighting can all drastically weaken its performance to the point of giving incorrect results and extracting errors.
- Challenges of Multilingual Content: Fintech companies work in a context that is considered global, and this often means that there are documents in various languages they have to deal with. Traditional template-based OCR systems, which normally rely on developing models for certain languages or scripts, are frequently hamstrung by their inability to extract this multilingual content. Font, Formatting, and Handwriting Differences: The fintech sector handles a large variety of document types, each with different fonts, format styles, and even handwritten components. Template-based OCR systems generally have difficulty identifying less common fonts, non-standard formats, or cursive handwriting.
- Strict Template Requirements: Template-based OCR systems require that a predefined template specifies separation properties, which include exact parameters for the dimensions of the document, the alignment of images, and text placement. Such a restriction means that it is less flexible to work with the dynamic and varied types of documents that finance and technology encounter.
Understanding the Technology Behind AI-Powered OCR
Machine Learning’s Role in OCR
- Supervised Learning: The models of AI are trained through powerful supervised label training on compressed data with characters, words, and layout. Then, they have to generalize and do well on unseen data.
- Neural Networks: Deep learning architecture, including Convolutional Neural Networks and Recurrent Neural Networks, is used for image and text sequence processing, thereby improving recognition accuracy.
- Transfer Learning: Fine-tuning of pre-trained models that were trained on large benchmark datasets such as ImageNet reduces the necessity to obtain training data for AI OCR tasks.
Advancements in Natural Language Processing (NLP)
- Contextual Understanding: NLP technologies give the OCR bots the ability to contextualize what the set of texts is about. These improvements make it a lot easier for text recognition to be resolved, especially for disoriented or little ambiguous inputs.
- Multilingual Support: NLP models are capable of handling multiple different languages and scripts, making AI OCR fit for applications all over the world.
- Semantic Analysis: Besides text extraction, OCR document verification can extract from the text meaningly semantic interpretations for some related applications like sentiment analysis, summarization, and data categorization.
Conclusion
AI-based OCR is revolutionizing the field of document verification, achieving unprecedented accuracy, efficiency, and versatility. This technology eliminates the shortcomings of traditional OCR systems in their incapacity for working with complex layouts, poor image quality, multilingual content, and diverse fonts and handwriting, thanks to advanced machine learning and natural language processing techniques.
FAQ:
What is OCR Technology?
OCR (Optical Character Recognition) is a technology used to convert printed or hand-written data to machine-readable digital data. OCR facilitates the reading of text from images, scanned reports, or PDFs, making storage, searching, and processing of data electronically easier.
How Optical Character Recognition Works?
Optical Character Recognition (OCR) functions by examining the shapes and patterns of characters found in an image or document. Initially, the image undergoes preprocessing to enhance its quality through adjustments in brightness, contrast, and resolution. The specification of a region of text within the image is determined by a means of pattern matching or machine learning detection of individual characters. After extraction, the text is passed on to the next process, where it will be spell-checked and formatted in a manner digestible by the computer.
What is the difference between OCR and AI?
OCR extracts text from images or documents through pattern recognition, whereas AI includes machine learning and natural language processing to perform tasks intelligently. AI improves OCR to read complex documents, learn from data, and enhance accuracy, forming AI-powered OCR.
What is AI-driven OCR Document Processing?
AI OCR document processing brings together conventional OCR and artificial intelligence to automate and improve document processing. It utilizes machine learning to identify complicated layouts, multilingual text, and varied fonts, and it can learn new types of documents without pre-defined templates.